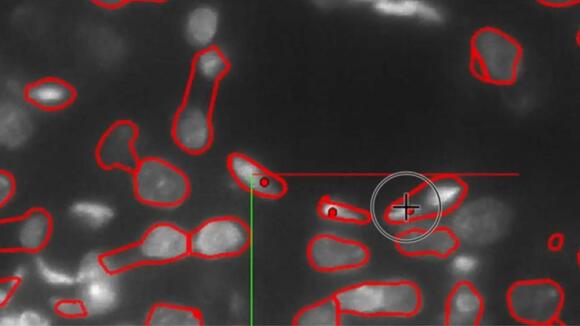
In a remarkable moment for Alzheimer’s disease research, researchers at the Massachusetts Institute of Technology (MIT) developed a Neuron Tracing and Active Learning Environment (NeuroTrALE) to better understand the disease.
Alzheimer’s is one of many debilitating neurological disorders that together affect one-eighth of the world’s population. While the new drug is a step in the right direction, there is still a long journey ahead to understand it fully and other such diseases.
“Reconstructing the intricacies of how the human brain functions on a cellular level is one of the biggest challenges in neuroscience,” says Lars Gjesteby, a technical staff member and algorithm developer from the MIT Lincoln Laboratory’s Human Health and Performance Systems Group.
“High-resolution, networked brain atlases can help improve our understanding of disorders by pinpointing differences between healthy and diseased brains. However, progress has been hindered by insufficient tools to visualize and process very large brain imaging datasets.”
Understanding the Brain Atlas
A networked brain atlas is a detailed map of the brain that can help link structural information to neural function. Brain imaging data need to be processed and annotated to build such atlases.
For example, each axon, or thin fiber connecting neurons , needs to be traced, measured, and labeled with information.
Current methods of processing brain imaging data, such as desktop-based software or manual-oriented tools, still need to be designed to handle human brain-scale datasets. As such, researchers often spend a lot of time slogging through an ocean of raw data.
NeuroTrALE is a software pipeline that brings machine learning, supercomputing, ease of use, and access to this brain mapping challenge.
NeuroTrALE automates much of the data processing and displays the output in an interactive interface that allows researchers to edit and manipulate the data to mark, filter, and search for specific patterns.
Machine-learning technique
One of NeuroTrALE’s defining features is the machine-learning technique it employs, called active learning.
NeuroTrALE’s algorithms are trained to automatically label incoming data based on existing brain imaging data, but unfamiliar data can present the potential for errors. Active learning allows users to manually correct errors, teaching the algorithm to improve the next time it encounters similar data.
This mix of automation and manual labeling ensures accurate data processing with a much smaller burden on the user.
“Imagine taking an X-ray of a ball of yarn. You’d see all these crisscrossed, overlapping lines,” said Michael Snyder from the laboratory’s Homeland Decision Support Systems Group.
“When two lines cross, does it mean one of the pieces of yarn is making a 90-degree bend, or is one going straight up and the other is going straight over? With NeuroTrALE’s active learning, users can trace these strands of yarn one or two times and train the algorithm to follow them correctly moving forward. Without NeuroTrALE, the user would have to trace the ball of yarn, or the axons of the human brain, every single time.” Snyder, along with staff member David Chavez, is a software developer on the NeuroTrALE team.
Because NeuroTrALE takes the bulk of the labeling burden off of the user, it allows researchers to process more data more quickly.
Further, the axon tracing algorithms harness parallel computing to distribute computations across multiple GPUs simultaneously, leading to even faster, scalable processing.
Using NeuroTrALE, the team demonstrated a 90 percent decrease in computing time needed to process 32 gigabytes of data over conventional AI methods.
The team also showed that a substantial increase in the volume of data does not translate to an equivalent increase in processing time.
Comments / 0