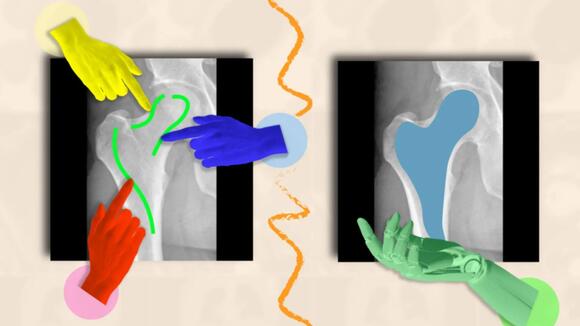
To the untrained eye, a medical image such as an MRI or X-ray may appear as a confusing collection of black-and-white shapes.
It can be challenging to distinguish where one structure, such as a tumor, ends and another begins.
When AI systems are trained to understand the boundaries of biological structures, they can segment (or delineate) regions of interest that doctors and biomedical workers want to monitor for diseases and other abnormalities.
Instead of wasting time manually tracing anatomy across multiple images, an artificial assistant could handle that task.
ScribblePrompt
Researchers from MIT’s Computer Science and Artificial Intelligence Laboratory (CSAIL), Massachusetts General Hospital (MGH), and Harvard Medical School have created an interactive tool called the “ScribblePrompt” framework.
This tool can quickly segment any medical image, even types it hasn’t seen before, without tedious data collection.
Instead of manually marking up each picture, the team simulated how users would annotate over 50,000 scans, including MRIs, ultrasounds, and photographs, across structures in the eyes, cells, brains, bones, skin, and more.
The team utilized algorithms to annotate all those scans, replicating how humans would annotate and click on various areas in medical images.
In addition to commonly labeled regions, the team utilized superpixel algorithms to identify potential new regions of interest for medical researchers and train ScribblePrompt to segment them.
ScribblePrompt has prepared synthetic data to handle real-world segmentation requests from users. This synthetic data will help ScribblePrompt to address real-world segmentation requests from users.
Reduces annotation time by 28%
“AI has significant potential in analyzing images and other high-dimensional data to help humans do things more productively,” said MIT PhD student Hallee Wong SM ’22, the lead author of a new paper about ScribblePrompt and a CSAIL affiliate.
“We want to augment, not replace, the efforts of medical workers through an interactive system. ScribblePrompt is a simple model with the efficiency to help doctors focus on the more interesting parts of their analysis. It’s faster and more accurate than comparable interactive segmentation methods, reducing annotation time by 28 percent compared to Meta’s Segment Anything Model (SAM) framework, for example.”
ScribblePrompt’s interface is simple: Users can scribble across the rough area they’d like segmented or click on it, and the tool will highlight the entire structure or background as requested.
For example, you can click on individual veins within a retinal (eye) scan. ScribblePrompt can also mark up a structure given a bounding box.
The tool can then make corrections based on the user’s feedback. For example, if you wanted to highlight a kidney in an ultrasound, you could use a bounding box and then scribble in additional parts of the structure if ScribblePrompt missed any edges.
If you wanted to edit your segment, you could use a “negative scribble” to exclude certain regions.
These self-correcting, interactive capabilities made ScribblePrompt the preferred tool among neuroimaging researchers at MGH in a user study.
93.8 percent of these users favored the MIT approach over the SAM baseline in improving its segments in response to scribble corrections.
As for click-based edits, 87.5 percent of the medical researchers preferred ScribblePrompt.
ScribblePrompt was trained on simulated scribbles and clicked on 54,000 images across 65 datasets featuring scans of the eyes, thorax, spine, cells, skin, abdominal muscles, neck, brain, bones, teeth, and lesions. The model familiarized itself with 16 medical images, including microscopies, CT scans, X-rays, MRIs, ultrasounds, and photographs.
“Many existing methods don’t respond well when users scribble across images because it’s hard to simulate such interactions in training. For ScribblePrompt, we were able to force our model to pay attention to different inputs using our synthetic segmentation tasks,” said Wong.
“We wanted to train what’s essentially a foundation model on a lot of diverse data so it would generalize to new types of images and tasks.”
Comments / 0