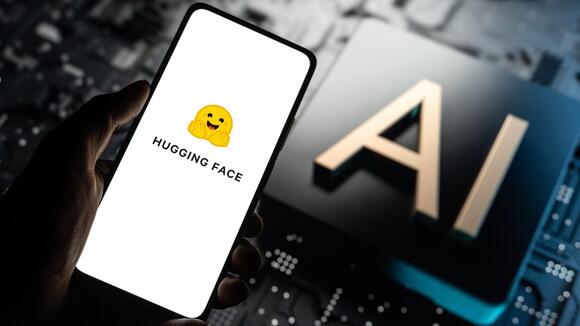
Hugging Face has released its second LLM leaderboard to rank the best language models it has tested. The new leaderboard seeks to be a more challenging uniform standard for testing open large language model (LLM) performance across a variety of tasks. Alibaba's Qwen models appear dominant in the leaderboard's inaugural rankings, taking three spots in the top ten.
Hugging Face's second leaderboard tests language models across four tasks: knowledge testing, reasoning on extremely long contexts, complex math abilities, and instruction following. Six benchmarks are used to test these qualities, with tests including solving 1,000-word murder mysteries, explaining PhD-level questions in layman's terms, and most daunting of all: high-school math equations. A full breakdown of the benchmarks used can be found on Hugging Face's blog .
The frontrunner of the new leaderboard is Qwen, Alibaba 's LLM, which takes 1st, 3rd, and 10th place with its handful of variants. Also showing up are Llama3-70B, Meta's LLM, and a handful of smaller open-source projects that managed to outperform the pack. Notably absent is any sign of ChatGPT; Hugging Face's leaderboard does not test closed-source models to ensure reproducibility of results.
Tests to qualify on the leaderboard are run exclusively on Hugging Face's own computers, which according to CEO Clem Delangue's Twitter, are powered by 300 Nvidia H100 GPUs. Because of Hugging Face's open-source and collaborative nature, anyone is free to submit new models for testing and admission on the leaderboard, with a new voting system prioritizing popular new entries for testing. The leaderboard can be filtered to show only a highlighted array of significant models to avoid a confusing glut of small LLMs.
As a pillar of the LLM space, Hugging Face has become a trusted source for LLM learning and community collaboration. After its first leaderboard was released last year as a means to compare and reproduce testing results from several established LLMs, the board quickly took off in popularity. Getting high ranks on the board became the goal of many developers, small and large, and as models have become generally stronger, 'smarter,' and optimized for the specific tests of the first leaderboard, its results have become less and less meaningful, hence the creation of a second variant.
Some LLMs, including newer variants of Meta's Llama, severely underperformed in the new leaderboard compared to their high marks in the first. This came from a trend of over-training LLMs only on the first leaderboard's benchmarks, leading to regressing in real-world performance. This regression of performance, thanks to hyperspecific and self-referential data, follows a trend of AI performance growing worse over time , proving once again as Google's AI answers have shown that LLM performance is only as good as its training data and that true artificial "intelligence" is still many, many years away.
Comments / 0