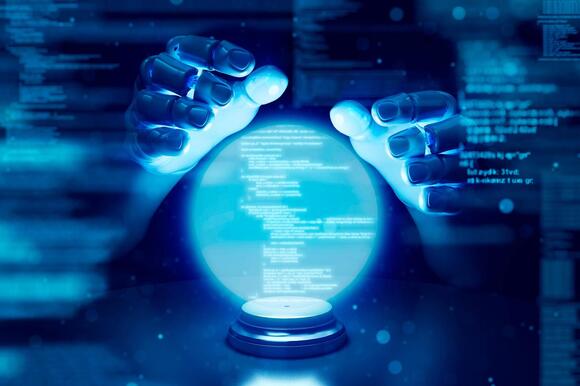
Whether playing the lottery or betting on a sporting event, everyone wishes they had a view into the future to see how things might turn out. Alas, that’s impossible.
Sportswear Industry Sees Solid Growth Ahead Despite Some Risks
But brands designing athletic wear like Public Rec and Athletic Propulsion Labs ( APL ) are doing what the can to get an informed read on consumers’ impending wants and needs. The companies have both turned to machine learning ( ML ) and artificial intelligence (AI) to solidify their inventory bets before they place them.
According to a report from forecasting firm WGSN, between 15 million and 45 million of the approximately 150 million garments produced annually never get sold. The company estimated that about 10 to 15 percent of that problem could be solved by stronger forecasting.
Justin Abrams, CEO and co-founder of early-stage startup Flagship RTL, has set out to help
companies on that front. The New York-based startup has already begun working with brands like Ministry of Supply, APL, Public Rec and Margaux. Its technology leverages ML and AI to more accurately forecast future seasons down to the SKU level, which means brands can gain specific insights on color, size and material attributes.
Abrams said he knows brands and retailers struggle with keeping the right products in stock and ensuring goods that may not be as popular don’t end up collecting dust in a warehouse—or worse, collecting dirt in a landfill.
Flagship RTL uses machine learning to ingest retailers’ historic sales data and uses it to
create forecasts brands and retailers can rely on. It also completes the process in a much shorter amount of time than it would take for a planner to crunch the same data.
“Because of the [lead] times you have to add, you need a real-time picture of the
future in order to make the best decision today. That’s hard enough if you have a few products; it’s impossible to get right if you have hundreds of thousands of products,” Abrams told Sourcing Journal. “We answer the question of how much to buy and when to hold it.”
Public Rec has already used that technology to forecast its upcoming season.
Cody Vidinich, chief product officer of the brand, said that while the company had previously used a different software tool, it had not been providing worthwhile results. Inventory, he said, has proven one of the Chicago-based brand’s biggest cost centers, which made turning to AI practical.
“Especially for us, [as a] high-growth startup, inventory is such a huge portion of what sits on our balance sheets, and if we’re over inventoried, that basically drains our ability to pay for marketing, which then slows our growth,” Vidinich said. “Being over inventoried is super dangerous, and then if we are paying for marketing and we’re not in stock, that’s just wasted spend. So nailing this inventory and minimizing out of stocks while minimizing overstock at the same time has been a huge priority for us.”
Abrams said in order to onboard customers using legacy methods for forecasting, the company has helped brands like Public Rec plan for upcoming seasons by issuing an Excel sheet indicating its ML system’s findings.
Once a client fully onboards onto the platform, that information is saved and available for the next forecasting request; Flagship can integrate data from other providers.
Abrams said the company had the confidence to build its software because of those Excel sheets. He wanted to avert the all-too-common startup struggle of building something customers don’t have trust in.
Manufacturers See Low Success Rates for Early Gen AI Initiatives
“We had yet to see brands fully adopt machine learning that exists on the market, so rather than build software in order to test, learn and eventually prove out adoption and trust in our forecast, we started surfacing ML model outputs in Excel spreadsheets,” he said. “Eventually, our customers started to adopt and trust our approach to forecasting and used [those Excel sheets] to make their inventory purchases.”
Vidinich said Public Rec used that Excel sheet to prove out the value of the solution and plan for next season. He said the abbreviated turnaround time for the forecast allowed the brand to plan for a shorter season than it usually would have.
“Typically, we’d be buying more like 26 weeks out or so—so, more at six-month chunks. We’d work to simplify the process by looking at a broader time period because [forecasting] was so manual to do,” he said. “Now, we’re getting into a place that we’re buying more like eight to 12 weeks out. I would expect our inventory turns to maybe cut in half—that may be a little aggressive, but it’s close.”
Other, larger brands have also begun migrating to Flagship. APL has not yet planned a season with the software, but already has thought through how the technology could help it keep the right balance of its top items in stock, said Ryan Goldston, co-CEO and cofounder of APL.
“For us, it [was]…about being proactive instead of reactive. When our core products sell out, we have a ‘notify me’ alert for customers. However, if we can reach a point where core products aren’t sold out as frequently due to Flagship’s technology, and we can forecast demand more precisely, that is a tremendous advantage,” Ryan Goldston told Sourcing Journal.
While the software automates recommendations, human planners can dive into the data to understand why the system offered up what it did. Noah Love, product engineer at Flagship, said the company wanted to make sure users didn’t feel shackled to the outputs and that they knew where the information had come from, rather than the technology operating in a black box.
That can help planners make decisions on items the company may feel more comfortable
with having out-of-stocks on. For instance, if a brand makes a seasonal color way of one of its core products, it may find that customers still purchase core colors when the bolder, limited-edition color runs out. But, if the customer comes for a core color and it’s out of stock, the brand may lose a sale.
Adam Goldston, APL’s other co-CEO and co-founder, said the team decided to partner with Flagship because it could hone in on specificities like that.
“Not all sizes sell at the same rate, and with the granularity of being able to assist at the style, color and size level, we see that as a great benefit that will help us as we continue to scale,” Adam Goldston said.
Love noted the platform does track what a human tweaked about Flagship’s recommendations, which helps train the model further—and can provide metrics about whether the human or the technology proved closer to being correct when sales numbers start coming in.
At large, the technology is meant to support human decision-making, not fully automate it,
Love said. In the time that teams save in building out nitty-gritty forecasts, they can shift their attention to the 10 to 20 percent of planning decisions that could make or break inventory strategy.
“The goal is to make more good decisions than bad ones. And just like a stock portfolio, you’ll have some winners and losers, but it’s about minimizing losses, maximizing gains efficiently and servicing the trends and data you need to make informed decisions without spending endless hours and resources,” Love said.
How Etsy Approaches AI--Both Internally and Externally
Comments / 0