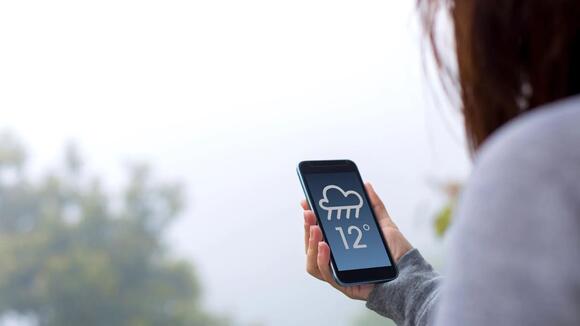
In a landmark achievement in forecasting weather accurately, Google researchers have developed a new machine learning (ML) based approach, called NeuralGCM, to simulate Earth’s atmosphere.
Developed with the European Centre for Medium-Range Weather Forecasts (ECMWF), NeuralGCM combines traditional physics-based modeling with ML for improved simulation accuracy and efficiency.
According to Google, this approach generates 2–15-day weather forecasts that are more accurate than the current gold-standard physics-based model and reproduces temperatures over a past 40-year period more accurately than traditional atmospheric models.
“Although we have not yet built NeuralGCM into a full climate model, it marks a significant step towards developing more powerful and accessible climate models,” Google said.
Physics and AI together
While new machine-learning techniques that predict weather by learning from years of past data are extremely fast and efficient, according to Google, they can need help with long-term predictions.
General circulation models, on the other hand, have dominated weather prediction for the last 50 years.
They use complex equations to model changes in the atmosphere and give accurate projections, but they are exceedingly slow and expensive to run.
Experts are divided on which tool will be most reliable in the future. However, Google’s new approach attempts to combine the two.
“It’s not sort of physics versus AI. It’s really physics and AI together,” Stephan Hoyer, an AI researcher at Google Research, said .
The new approach uses a conventional model to work out some of the large atmospheric changes required to make a prediction.
It then incorporates AI , which tends to do well where those larger models fall flat—typically for predictions on scales smaller than 25 kilometers, like those dealing with cloud formations or regional microclimates.
“That’s where we inject AI very selectively to correct the errors that accumulate on small scales,” Hoyer said.
Climate modeling with NeuralGCM
According to Google, although traditional climate models have improved over the decades, they often generate errors and biases due to scientists’ incomplete understanding of Earth’s climate and how the models are built.
The traditional models divide the globe into cubes—typically 50–100 km on each horizontal side—that extend from the surface up into the atmosphere and then predict what happens to the weather in each cube over a period of time.
To make predictions, they calculate how air and moisture move based on well-established laws of physics.
However, many important climate processes, including clouds and precipitation, vary over much smaller scales (millimeters to kilometers) than the cube dimensions used in current models and, therefore, cannot be calculated based on physics.
Scientists also need a complete physical understanding of some processes, such as cloud formation.
These traditional models don’t rely on first principles alone. Instead, they use simplified models to generate approximations, called parameterizations , to simulate small-scale and less understood processes.
These simplified approximations inherently limit the accuracy of physics-based climate models.
Like a traditional model, NeuralGCM divides the Earth’s atmosphere into cubes and runs calculations on the physics of large-scale processes like air and moisture movement.
But instead of depending on parameterizations formulated by scientists to simulate small-scale aspects like cloud formation, it uses a neural network to learn the physics of those events from existing weather data.
Google claimed that it trained a suite of NeuralGCM models using weather data from ECMWF from 1979 to 2019 at 0.7°, 1.4°, and 2.8° resolution.
“Although our models were trained on weather forecasts, we designed NeuralGCM to be a general-purpose atmospheric model,” Google said.
Comments / 0